Generative AI has been around for decades, but the systems exploded into the public consciousness in 2022, when OpenAI released ChatGPT, an AI chatbot that could produce remarkably human-like text.
The AI gained this ability by analyzing a lot of text created by people, mostly pulled from the internet. To put it simply, from this data, it learned to predict what word was most likely to come next in a sequence based on the words that came before it.
To improve their generative AIs, OpenAI and other developers need ever more high-quality training data — but now that publishers know their content is being used to train AIs, they’ve started requesting money for it and, in some cases, suing developers for using it without permission.
Even if developers had free access to all the data online, though, it still wouldn’t be enough.
“If you could get all the data that you needed off the web, that would be fantastic,” Aidan Gomez, CEO of AI startup Cohere, told the Financial Times. “In reality, the web is so noisy and messy that it’s not really representative of the data that you want. The web just doesn’t do everything we need.”
Cohere, OpenAI, and other AI developers think “synthetic data” — content generated by an AI, rather than humans — may be able to solve this problem.
On the surface, this does seem like a great idea: just have your current generative AI churn out loads of text, images, or videos — whatever you need — and then use that new additional data to train your new model. No need to worry about running out of content or running up against the demands of content creators.
It’s not quite that simple, though.
In a new paper published in Nature, a team of British and Canadian researchers fine-tuned a pre-trained large language model (LLM) — the kind of AI behind ChatGPT — on a dataset of Wikipedia articles.
They then pulled a segment of text from the training dataset (the Wikipedia articles) and prompted their fine-tuned LLM to predict the next bit of text. They repeated this process until they had a trove of synthetic data as large as the original Wikipedia dataset.
They then fed the synthetic data back into training the model and repeated the process, fine-tuning the AI and then using it to generate more synthetic data for training. After nine rounds of this recursive training, the AI was producing pure gibberish.
Here’s an example of a prompt and what the AI produced in response initially and after nine rounds of training on AI-generated data:
Input: some started before 1360 — was typically accomplished by a master mason and a small team of itinerant masons, supplemented by local parish labourers, according to Poyntz Wright. But other authors reject this model, suggesting instead that leading architects designed the parish church towers based on early examples of Perpendicular.
Output of Gen 0: Revival architecture such as St. John’s Cathedral in London. The earliest surviving example of Perpendicular Revival architecture is found in the 18th @-@ century Church of Our Lady of Guernsey, which dates from the late 19th century. There are two types of perpendicular churches : those.
Output of Gen 9: architecture. In addition to being home to some of the world’s largest populations of black @-@ tailed jackrabbits, white @-@ tailed jackrabbits, blue @-@ tailed jackrabbits, red @-@ tailed jackrabbits, yellow @-.
Co-author Ilia Shumailov told Freethink the problem is that the first synthetic data generated by the LLM contained mistakes. Feeding that flawed text to the AI caused it to produce more errors the next time around, and on and on.
He compares it to repeatedly scanning a picture, printing the file, and then scanning that picture: “In this process, scanner and printer will keep on adding errors, ultimately producing something that no longer looks like the original image. Same happens in [machine learning].”
Shumailov told Freethink that this issue, which his team calls “model collapse,” applies to any kind of generative AI trained on synthetic data, not just LLMs. Other studies focused on image-generating AIs seem to prove his point.
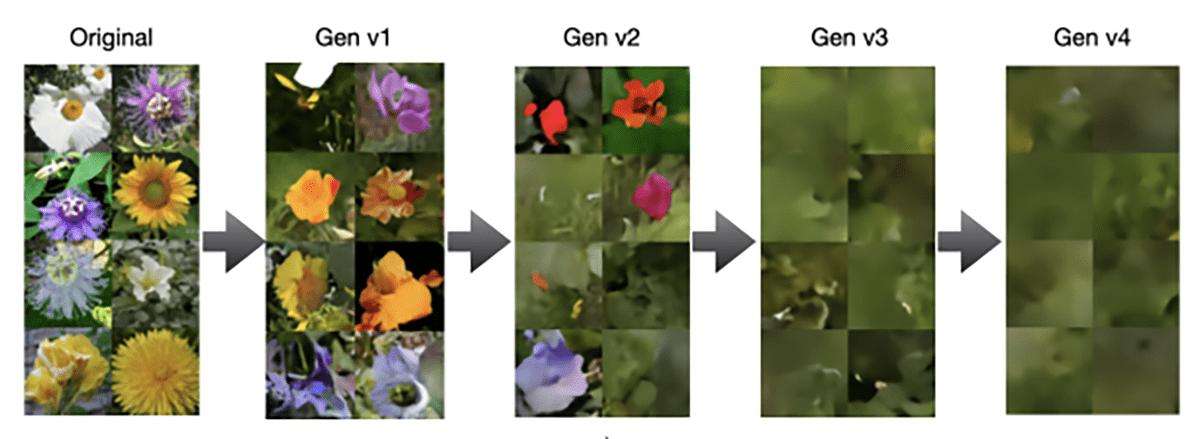
Synthetic data isn’t the only possible source of new training material for generative AIs — OpenAI reportedly went the controversial route of transcribing more than one million hours of YouTube videos to feed its text models — but avoiding it might prove impossible.
Although generative AIs are relatively new, their content is quickly spreading across the web, and some experts think that a majority of content on the internet could be AI-generated just a few years from now.
This means even if AI developers don’t actively seek out synthetic data for training, models that have access to the internet might still ingest it alongside human-created content.
“The open question for researchers and companies building AI systems is: how much synthetic data is too much,” Jathan Sadowski, a lecturer in emerging technologies at Monash University, told AFP.
It’s possible that allowing even a little synthetic data into an AI’s diet could have a negative effect on its output.
Generative AIs are essentially probability machines — you submit a prompt, and they respond with the text or image they think is most likely to meet the brief. To improve their chances of being right, they might pass over options that would make sense, but that aren’t necessarily the most obvious answers, in favor of seemingly sure things.
Emily Wenger, an assistant professor of electrical and computer engineering at Duke University, used the example of asking a generative AI to produce images of dogs to demonstrate how this could affect AIs trained on synthetic data.
“The AI model will gravitate towards recreating the breeds of dog most common in its training data, so might over-represent the Golden Retriever compared with the Petit Basset Griffon Vendéen, given the relative prevalence of the two breeds,” she wrote in Nature.
Feed the AI its own dog images enough times, and eventually, errors in those will prevent it from being able to produce images that look like dogs at all. Before that happens, though, you’ll reach a point where it only generates images of Golden Retrievers if asked for pictures of dogs.
In practice, this means training AIs on any amount of synthetic data could make them more likely to produce biased, flawed content, even if it’s not enough to trigger complete model collapse.
Generative AI developers are now scrambling to figure out solutions to these problems.
The creation of advanced AI detection tools and regulations requiring labels on AI-generated content could help keep it out of training datasets, but some would surely still slip through the cracks and that doesn’t solve the problem of needing more high-quality training data.
Having humans or even other AIs evaluate synthetic data before it’s used for training could improve its quality, but it’s not clear how scalable that would be — people need to be paid and AIs are expensive to run.
Ultimately, no one knows for sure what the answer to the problem will be, but given how quickly AI-generated “slop” is filling up the internet, developers are going to need to figure it out — fast.
We’d love to hear from you! If you have a comment about this article or if you have a tip for a future Freethink story, please email us at [email protected].